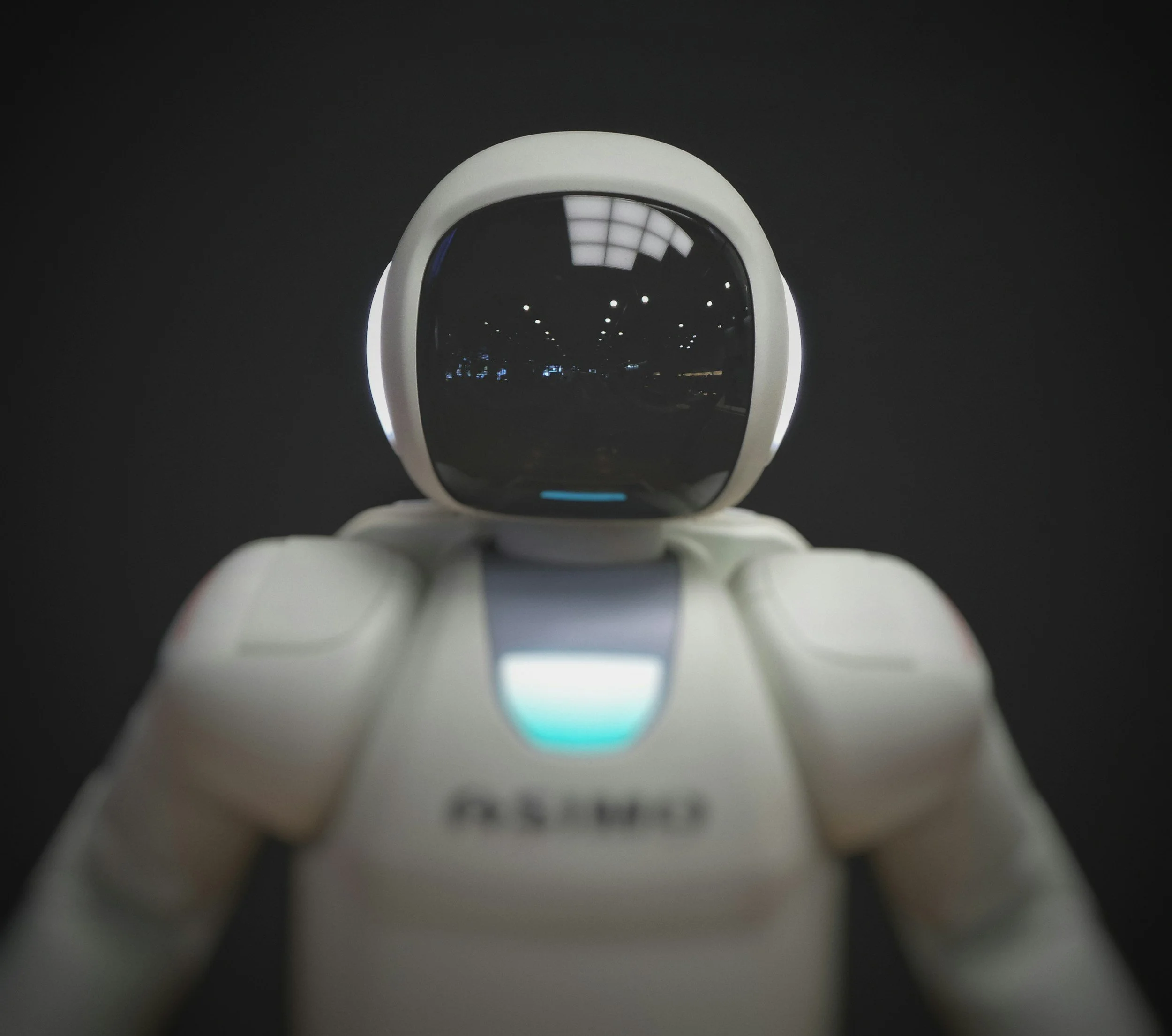
The Road Ahead: What if AI Delivers on its Potential?
In recent months, public discourse around artificial intelligence has tilted sharply toward skepticism. From headlines warning of a looming "AI bubble" to Reddit threads lamenting overvaluation and hype, it seems many have lost faith in what AI can actually deliver. Hype or bust?
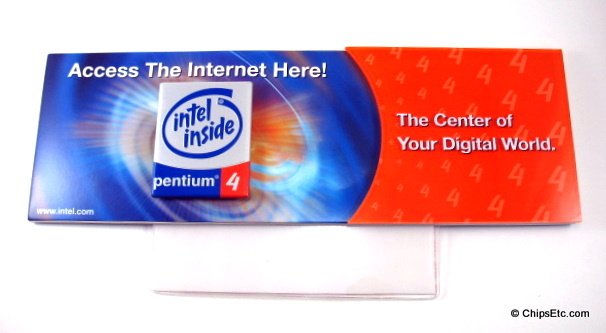
Deepseek and OpenAI is Just the Tip of an Iceberg
How will AI be shaped by the forces at hand? How do we predict how AI will look like in 5 years from now? AI, much like other transformative technologies, does not develop in isolation. What we see—the outputs of AI models, advancements, and regulations—is merely the tip of the iceberg. Beneath the surface, deeper patterns, policies, and ideologies are driving the trajectory of AI, shaping how nations invest in, control, and apply the technology.
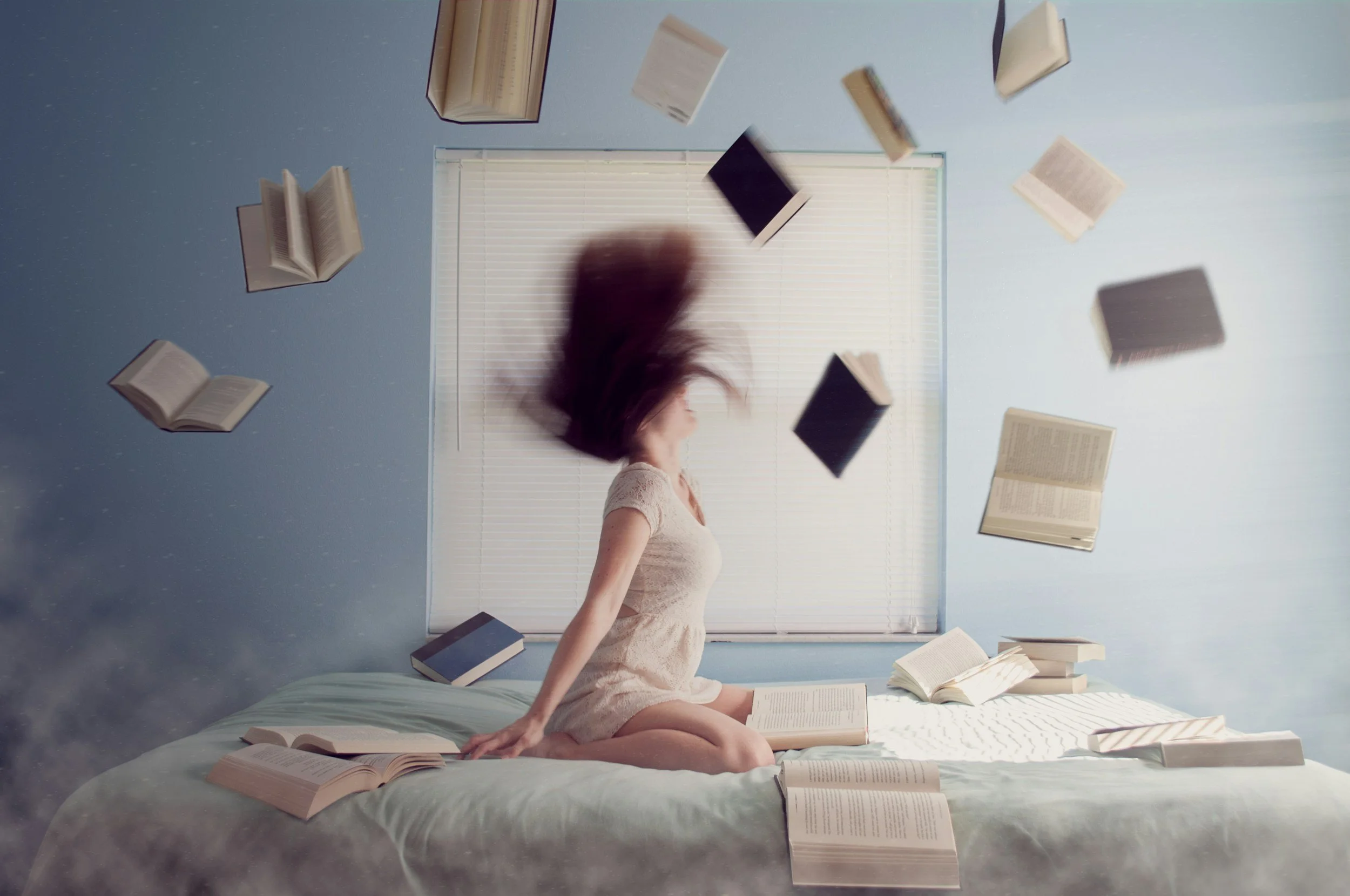
Teaching Students to Learn Effectively: A Cognitive Science Starter Guide for Educators
Teaching students how to learn is just as important as teaching them what to learn and when to learn. Effective study strategies can transform a student’s academic experience, turning frustration into mastery. One such evidence-based strategy is the SOAR method—Select, Organize, Associate, Regulate. Grounded in research…
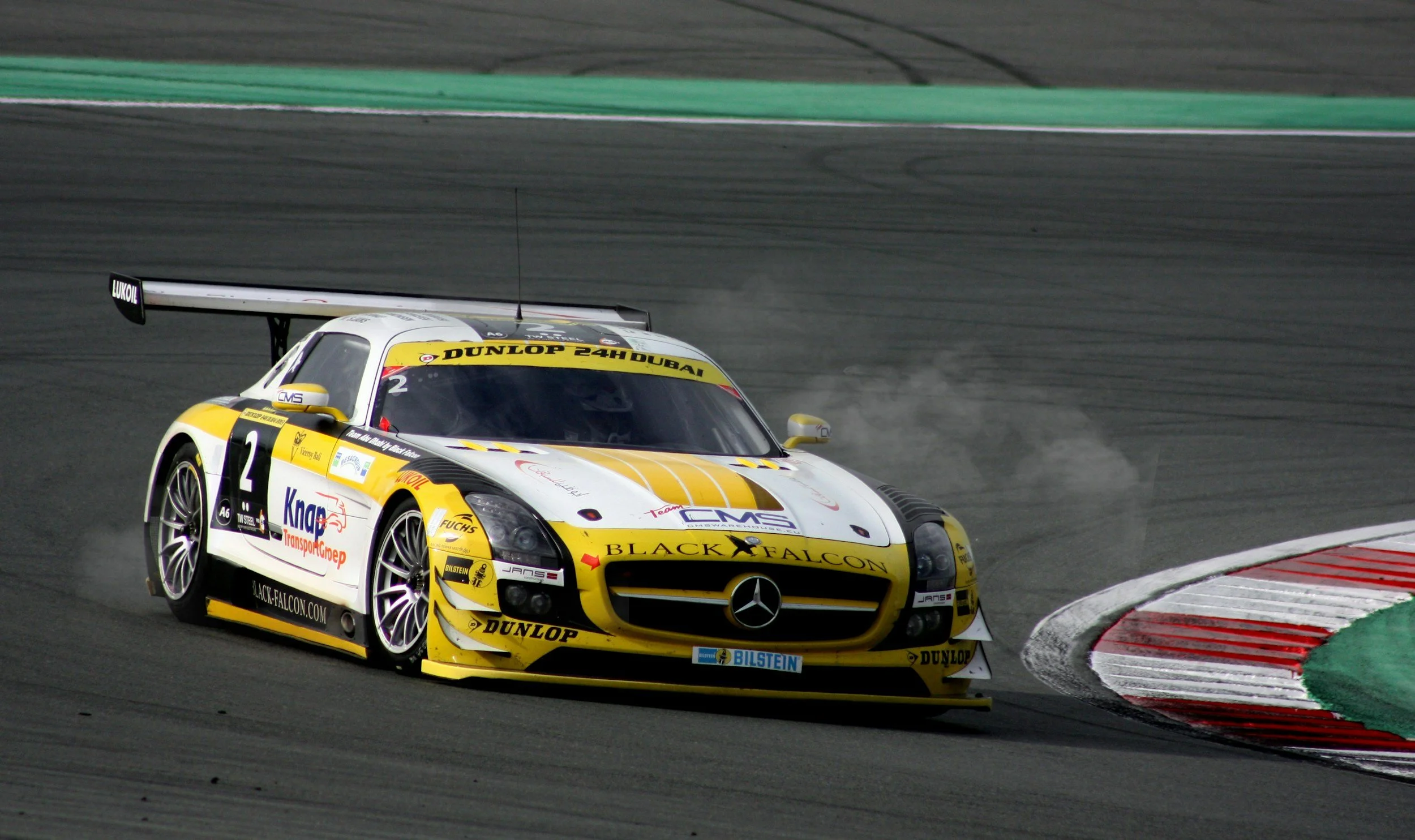
The Race for the Killer APP in Gen AI
Over the past three years, the AI landscape has witnessed a surge in innovation and investment. Thousands of AI startups have emerged globally, powered by over $330 billion in funding and an influx of venture capitalists looking to harness the transformative power of generative AI and large language models (LLMs). The industry has seen impressive advancements, particularly in content generation and customer service automation, but one key element remains elusive—the “killer app.” Despite this massive wave of AI startups, roughly 90% have struggled to achieve long-term success, largely due to challenges in scaling, finding product-market fit, and sustaining funding
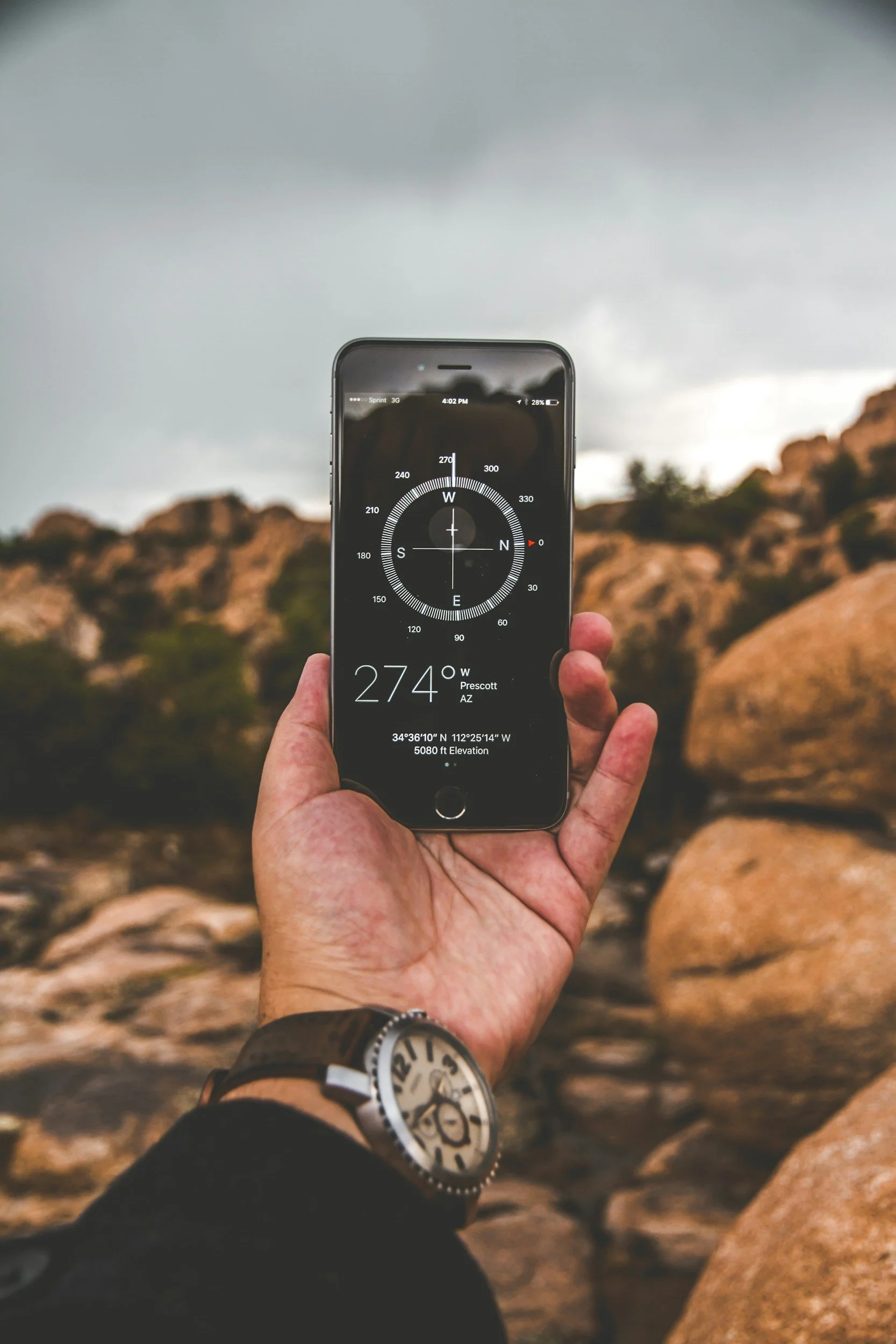
How Vector Databases and Knowledge Packs Are Transforming Data Retrieval and AI Interaction
As we move further into an era dominated by vast amounts of unstructured data, new methods are needed to make this information useful, accurate, and accessible. Vector databases and knowledge packs—a concept that enables large language models (LLMs) to engage with unstructured data—are pioneering this transformation, allowing AI to understand and respond based on the true meaning of content. Together, these innovations are changing how we store, retrieve, and utilize information across industries.
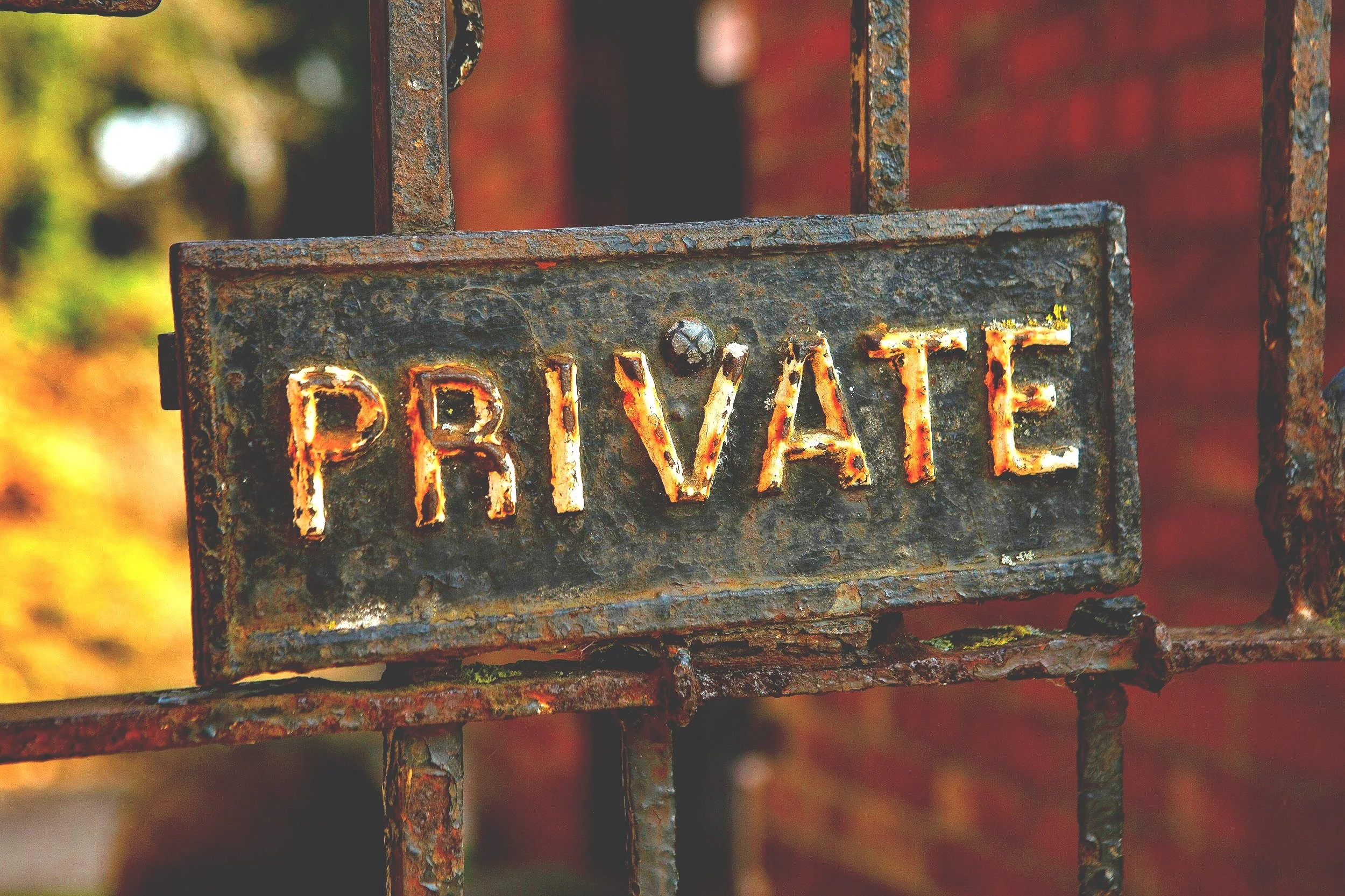
The Future of AI Agents: How Privacy-Centric Retrieval-Augmented Generation (RAG) Could Redefine Contextual Intelligence
Imagine an AI assistant that could dive into your personal calendar, seamlessly retrieve confidential documents, and deliver real-time financial insights—all while safeguarding your data privacy. For many, this sounds like the next frontier of artificial intelligence: AI agents empowered by Retrieval-Augmented Generation (RAG). RAG has surged to the forefront of AI technology by enabling intelligent agents to pull in real-time data and offer contextually relevant, informed responses.
But as powerful as RAG is, much of the data that makes it truly valuable—patient records, purchase histories, employee data, and more—remains private. In a world increasingly defined by data privacy concerns, RAG’s future success hinges on its ability to balance innovation with robust data protection measures. This shift is turning attention toward privacy-first RAG applications that keep personal and sensitive data secure while delivering unparalleled contextual intelligence.

Understanding Agentic AI: Origins, Architecture, and Integration with Large Language Models
The term "agentic AI" underscores a significant shift in artificial intelligence from passive systems to active agents capable of autonomous decision-making and action, as evidenced by the evolution of Large Language Models like GPT-o1 and Llama, Claude etc. This transformation involves integrating LLMs with diverse technologies, enabling them to operate independently and interact with their environments effectively. Such advancements raise critical safety, ethical, and alignment challenges, necessitating robust control mechanisms and transparency. Agentic AI is increasingly sought after for its ability to enhance productivity and automate complex tasks, offering scalable solutions across various sectors. These developments, combined with societal and cultural influences, are fueling public interest and regulatory focus, driving both academic and commercial investments to explore and mitigate the complexities and risks associated with these autonomous systems.
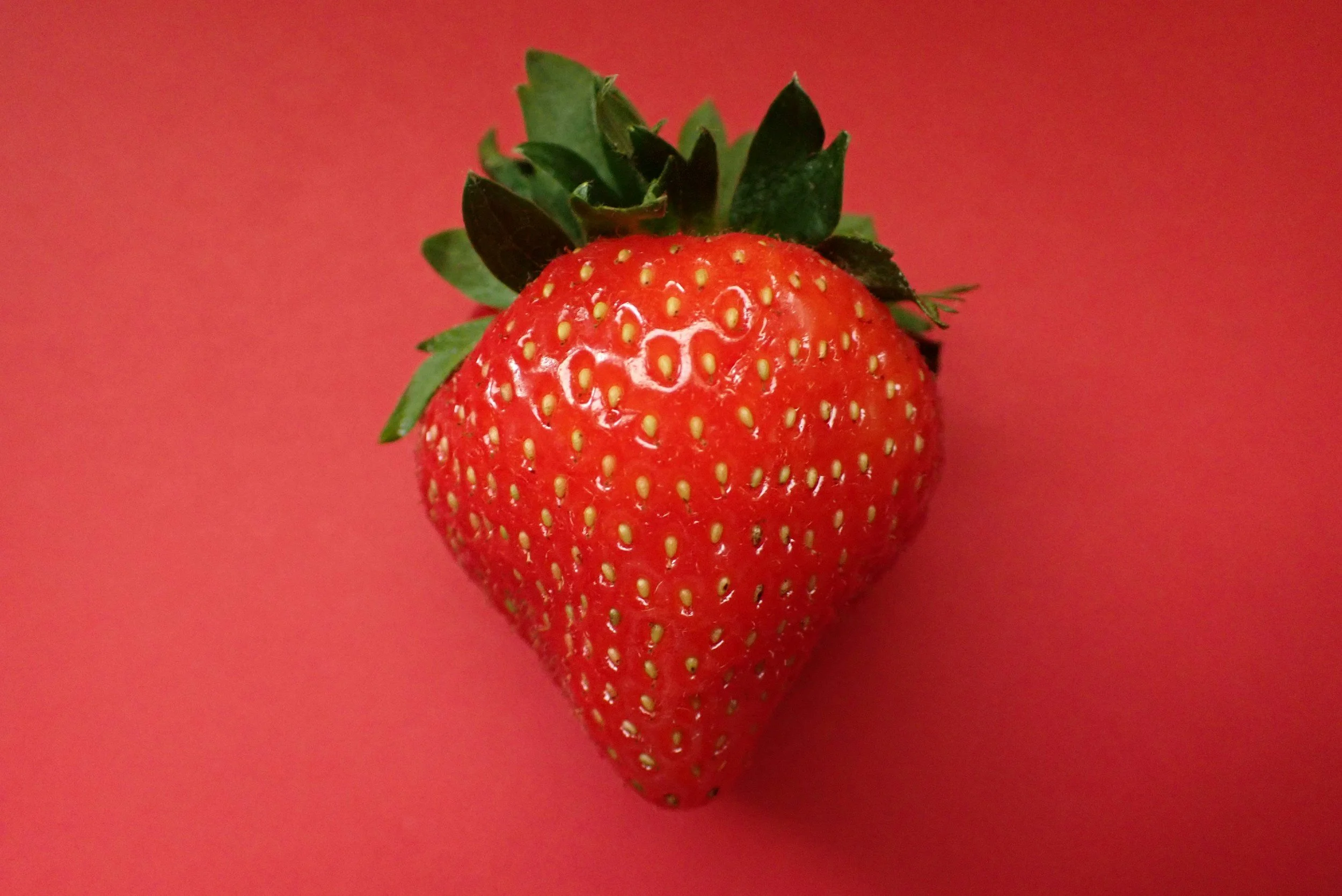
Is the Latest Release of OpenAI’s Strawberry as Sweet as It’s Set Out To Be?
Is OpenAI's latest model, Strawberry (o1), as sweet as it sounds? Touted as a game-changer in AI reasoning, it promises to solve complex problems in math, coding, and science with unprecedented accuracy. But with mixed reactions about its broader use and ethical concerns surrounding transparency, there's more to this story than just its technical prowess. Dive in to explore whether Strawberry truly delivers or if it comes with some bitter notes.
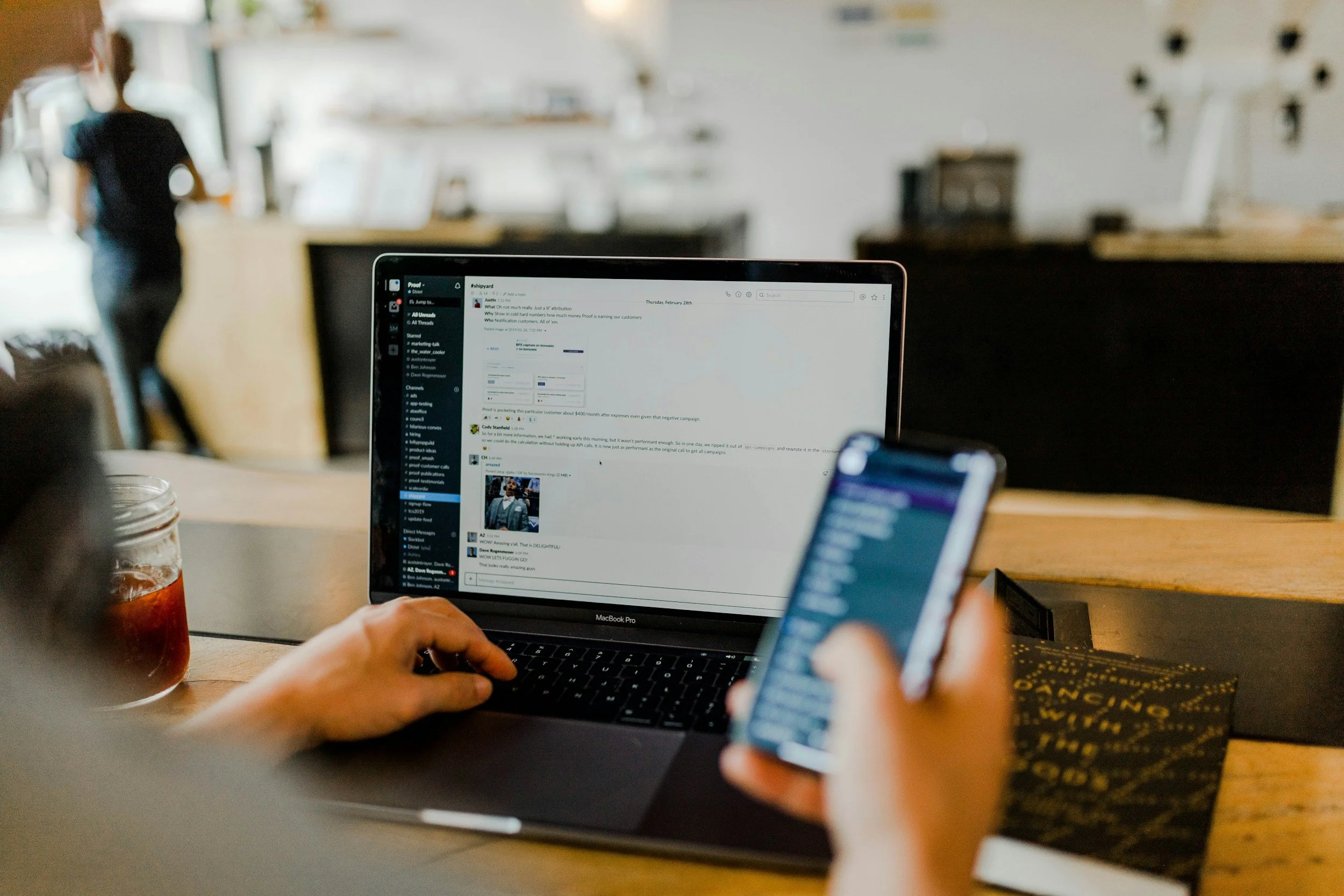
Cognitive Labor: The Hidden “Tax” on Business Productivity and How AI Can Minimize It
In today's knowledge-driven economy, businesses rely on skilled workers to solve problems, innovate, and make strategic decisions. However, a significant portion of these knowledge workers' time is consumed by repetitive, routine tasks that don’t fully utilize their expertise. This burden—what we can think of as cognitive labor—acts like a tax on a company's productivity and profitability. Much like actual taxes, cognitive labor is a cost businesses must pay, but with the right tools, it can be minimized.
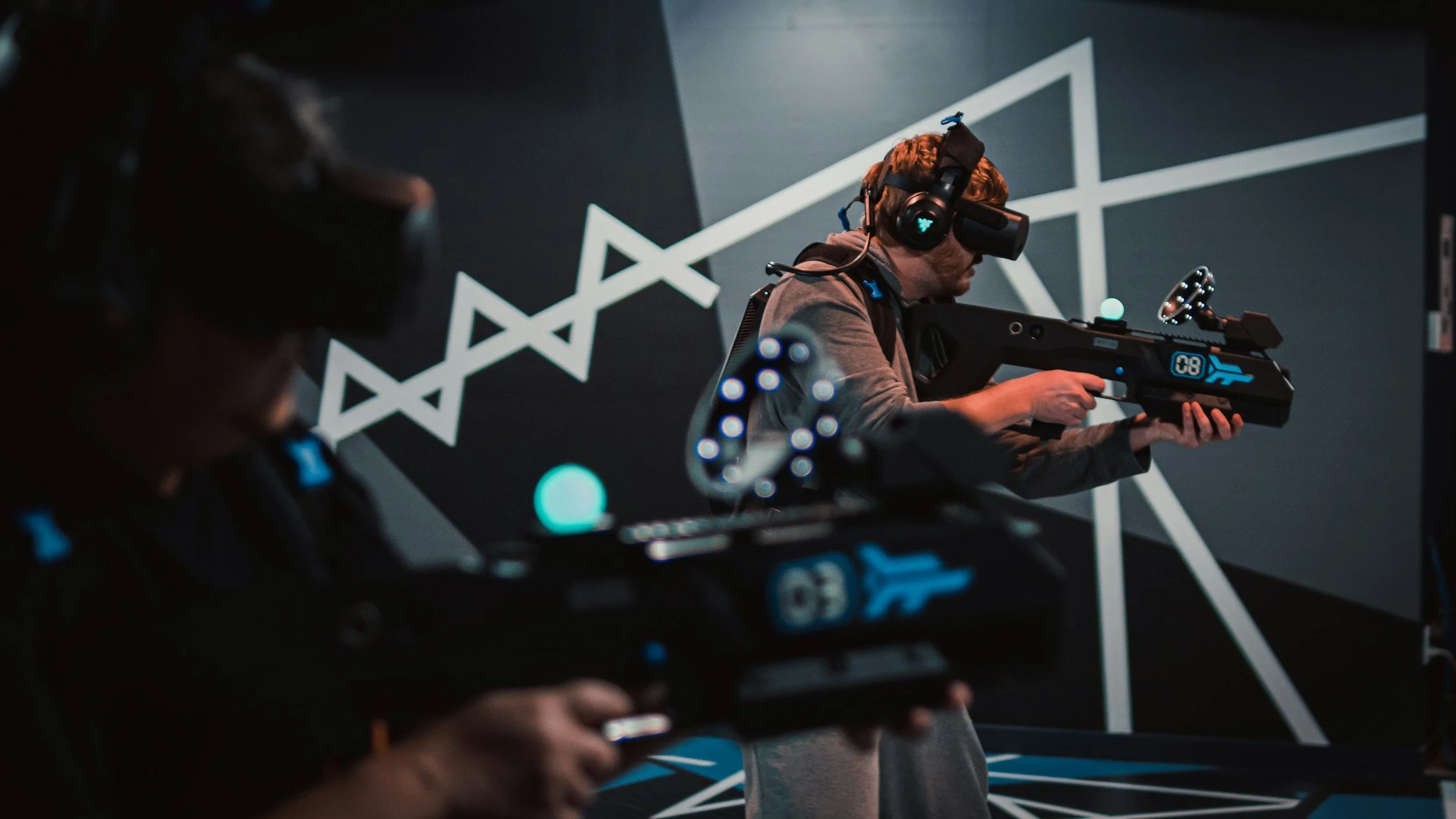
The Maturing State of the Metaverse: A 2024 Perspective
Is the metaverse dead? Not at all. While the initial hype has faded, the metaverse is evolving—fueled by advancements in AI, augmented reality (AR), and virtual reality (VR). Companies like Microsoft and Apple are leading this transformation with practical applications in enterprise, healthcare, and retail. Microsoft’s HoloLens 2 and Apple’s Vision Pro are reshaping how we work, learn, and interact, blending the digital and physical worlds in powerful new ways. If you thought the metaverse was just a passing trend, it’s time to take a second look. Its journey is just beginning.